In 2005, Stanford epidemiologist John Ioannidis published the provocatively titled paper “Why most published research findings are false” (Ioannidis, PLoS Med 2005, 2:e12), that has since become a foundational piece of metascience. Among other things, he stated:
The smaller the study sample conducted in a scientific field, the less likely the research findings are to be true.
The smaller the effect sizes in a scientific field, the less likely the research findings are to be true.
The greater the number and the lesser the selection of tested relationships in a scientific field, the less likely the research findings are to be true.
The greater the financial and other interests and prejudices in a scientific field, the less likely the research findings are to be true.
Ioannidis’ conclusions were hotly debated, and rejected by some. Nonetheless, his provocative wording aside, he touched a nerve, and succeeded in bringing the reproducibility crisis in medicine into the open.
Of course these concerns are not new. Already in 1962, Jacob Cohen published a study of psychology papers from 1960, finding that the average study had a power of only 0.48 to detect a medium sized (0.5 SD difference) effect (Cohen, J Abnorm Soc Psychol 1962, 65:145-153). And in a 1994 editorial in BMJ, Oxford statistician (and Equator co-founder) Doug Altman lamented “The scandal of poor medical research”:
“What should we think about a doctor who uses the wrong treatment, either willfully or through ignorance, or who uses the right treatment wrongly (such as by giving the wrong dose of a drug)? Most people would agree that such behaviour was unprofessional, arguably unethical, and certainly unacceptable. What, then, should we think about researchers who use the wrong techniques (either willfully or in ignorance), use the right techniques wrongly, misinterpret their results, report their results selectively, cite the literature selectively, and draw unjustified conclusions? We should be appalled. Yet numerous studies of the medical literature, in both general and specialist journals, have shown that all of the above phenomena are common. This is surely a scandal.”(Altman, BMJ 1994, 308:283-284)
Pharma Blues
Not only is clinical medicine in trouble, so is bench research. A study by the pharmaceutical company Bayer [eod_ticker target=”BAYN.XETRA”] (Prinz, Nat Rev Drug Discov 2011, 10:712) revealed that only 20-25% studies could be reproduced:
“Surprisingly, even publications in prestigious journals or from several independent groups did not ensure reproducibility. Indeed, our analysis revealed that the reproducibility of published data did not significantly correlate with journal impact factors, the number of publications on the respective target or the number of independent groups that authored the publications.”
“incorrect or inappropriate statistical analysis of results or insufficient sample sizes, which result in potentially high numbers of irreproducible or even false results, have been discussed”
Bayer is not alone. The US pharma company Amgen [eod_ticker target=”AMGN.US”] could replicate only 11% (6 of 53) of highly promising (‘landmark’) results they investigated in Hem-Onc. Even more worrisome was their finding that some of the research has resulted in clinical studies, suggesting patients were subjected to an experimental regimen that probably wouldn’t work (Begley, Nature 2012, 483:531-533).
The venture capital world has also noticed the strange inability to replicate results, and apparently they apply a 50% discount to published findings
“at least 50% of the studies published even in top tier academic journals – Science, Nature, Cell, PNAS, etc… – can’t be repeated with the same conclusions by an industrial lab.”
(LifeSciVC is a blog worth following, by the way)
How Did We Get Here?
The explanation is, unsurprisingly, multifactorial. Underpowered studies, the pervasive practice of multiple comparisons, p-hacking, and perverse incentives as part of the “publish or perish” culture, all play a part.
UNDERPOWERED STUDIES. I already mentioned Jacob Cohen’s 1962 paper finding that the average psychology study had a power of only 0.48 to detect a medium sized (0.5 SD difference) effect (Cohen, J Abnorm Soc Psychol 1962, 65:145-153). Later publications found that, for example 80% of RCTs that reported negative results didn’t collect enough data to detect a 25% difference, and nearly two-thirds were not powered up to detect even a 50% difference. (Moher, JAMA 1994, 272:122-124)
A review of all negative phase III oncology trials reported at ASCO found that 45% were underpowered to detect even a large difference (OR/HR ≥ 2). Only 11% of negative studies were powered to detect a small difference (OR/HR ≥ 1.3) and fewer than 10% of all studies explained why their samples were so small. (Bedard, J Clin Oncol 2007, 25:3482-3487)
MULTIPLE COMPARISONS. The average study includes not one but many tests, so it has a good chance of finding something significant — and a determined researcher will find significance. A review of 67 medical trials in the 1980s found that the average study made 30 comparisons; 75 % had the statistical significance of at least one comparison impaired by the problem of multiple comparisons, and 22% had the statistical significance of all comparisons impaired by the problem of multiple comparisons. (Smith, Am J Med 1987, 83:545-550). The probability of false positives rises exponentially with the number of comparisons
:
The multiple-comparison problems is humorously illustrated in this xkcd com comic:
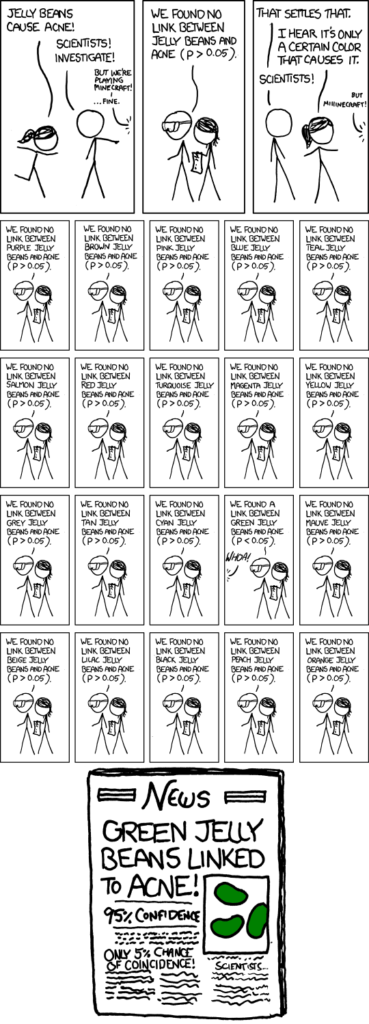
P-HACKING. Even more concerning was a finding that many published psychology papers report p-values that cluster implausibly around 0.05, as if researchers looked for significance until they found it. (Simonsohn, J Exp Psychol Gen 2014, 143:534-547). This “I am just looking for interesting” (a.k.a. significant to p<0.05) associations is a very popular, albeit dishonest, activity variously referred to as p-hacking, data dredging, or -fishing, and is central to the rot in medical research. You know you’ve made it when Urban Dictionary has an entry for your pastime:
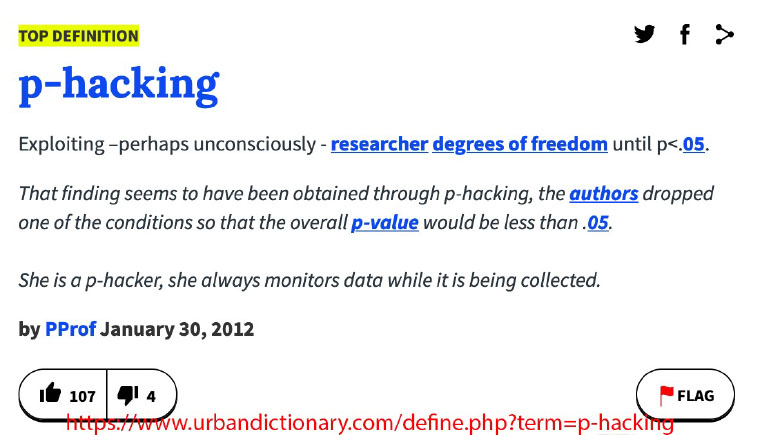
I really enjoy the tongue in cheek mention to “researcher degrees of freedom,” by the way.
Other explanations include:
- infrequent preregistration of studies. Journals could request preregistration of the study methods and target variables to prevent post-hoc data dredging. Although clinicaltrials.gov is a step in the right direction, the majority of published studies are still not preregistered.
- perverse incentives as part of the academic system of promotion, exemplified by the dictum “Publish [at all cost, no matter what trash] or perish”. Replacing the raw number of papers with the h-index (PNAS 2005, 102:16569) is a step in the right direction, but is far from sufficient: whereas in physics an h-index of 12 might be a typical value for promotion to associate professor (and 18 for advancement to full professor), in medicine I notice a phenomenon of h-index inflation. Nonetheless, evaluating the impact of the publication as opposed to its mere release upon an unsuspecting universe, through whatever means, is a necessary, but not sufficient step.
- a fragmented research landscape, in which individual groups have access to only small datasets;
- lack of transparent sharing of datasets and data analysis procedures. In addition to preregistration, journals should require sharing the data set (stripped of patient identifiers) and analysis procedures (as an R Notebook or similar markdown document).
- publication bias. Simply put, editors like being the first to publish positive findings. Being FIRST!! and BIG!!! really helps. Lay journalists, even less knowledgeable about the scientific method, basic data science, and the conduct of research, and working in an environment largely devoid of professional standards, then compound the problem with their “260% more dangerous!!!!” or similar cries. Unfortunately, early and positive findings are inconvenienced by the problem of the regression to the mean; hence their irreproducibility. It is worth noting that preregistration might mitigate this problem to some extent, but negative findings should also be published regularly, because they are at least as useful as their preferred positive counterparts. I illustrate this bias in the following cartoon:
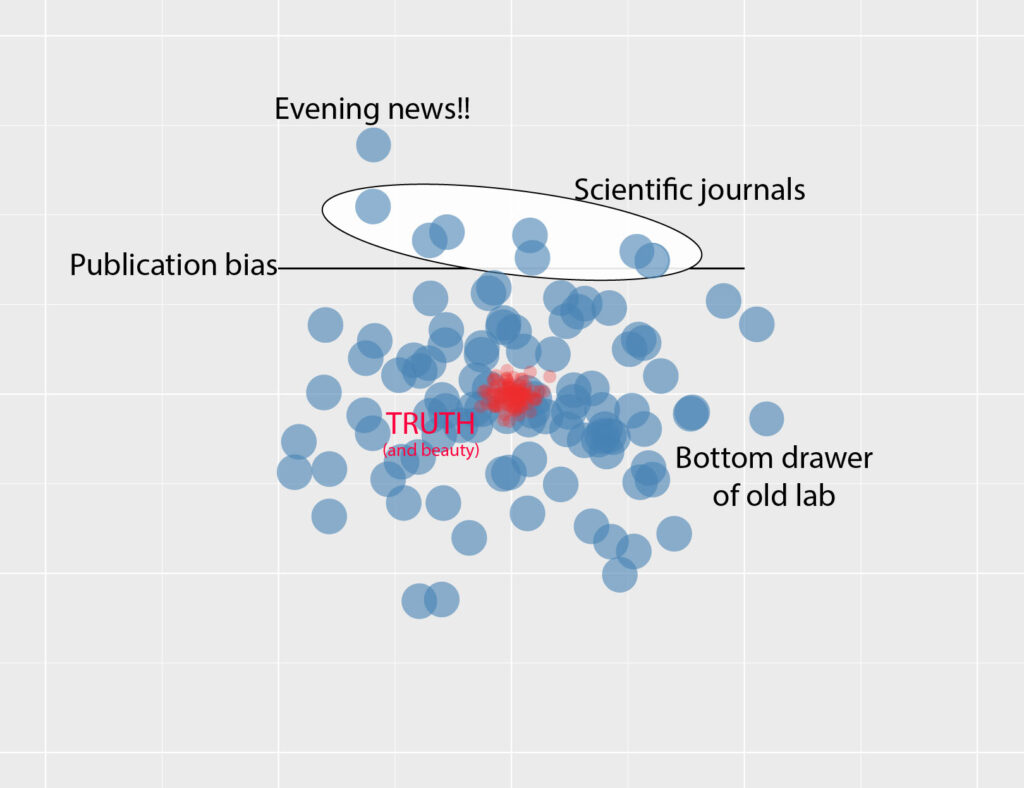
How to fix this mess
Alerted to the problem of NHST, and more specifically p-hacking, the ASA put out a statement (Wasserstein, American Statistician 2016, 70:129-131) on the use and the limitations of the p-value:
- P-values can indicate how incompatible the data are with a specified statistical model.
- P-values do not measure the probability that the studied hypothesis is true, or the probability that the data were produced by random chance alone.
- Scientific conclusions and business or policy decisions should not be based only on whether a p-value passes a specific threshold.
- Proper inference requires full reporting and transparency
- A p-value, or statistical significance, does not measure the size of an effect or the importance of a result.
- By itself, a p-value does not provide a good measure of evidence regarding a model or hypothesis.
They introduced it with the following anecdote:

Other groups, not satisfied by the mere enunciation of best practice standards, proposed reducing the p-value threshold to 0.005 (Benjamin, Nat Hum Behav 2018, 2:6-10). Reducing the p-value to 0.005 would increase the Bayes factor of the given analysis from the current value around 3 to around 20, while requiring a sample size increase by ~70% to maintain 80% power, but fundamentally I think will not address the core problem of NHST.
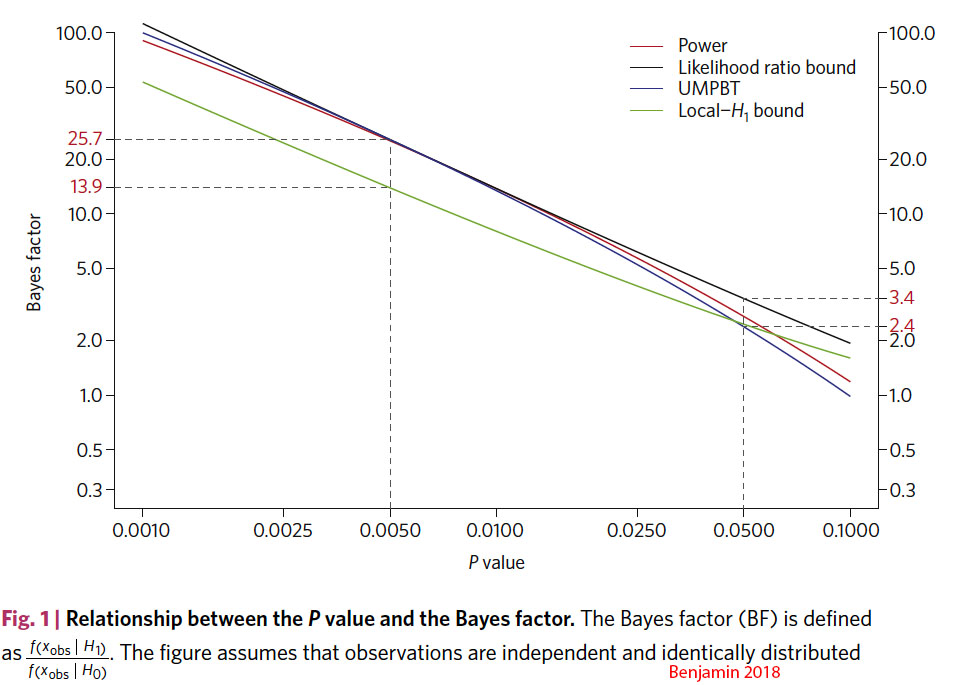
Others, noting that statistical significance can be obtained from pure noise, propose abandoning NHST testing altogether (McShane, American Statistician 2019, 73:235-245). Instead, they favor treating the p-value continuously, and considering it along with the currently subordinate factors (prior evidence, plausibility of mechanism, study design and data quality, real world costs and benefits, novelty of finding, and other factors that vary by research domain) as just one among many pieces of evidence.
Final thoughts
Richard Royall is quoted as having once said that there are three questions at the heart of science:
- ‘What is the evidence?’
- ’What should I believe?’
- ’What should I do?’
And he notes that any one single method cannot answer all these questions (Nuzzo, Nature 2014, 506:150-152). McShane et al observed that biology in general, and the part that underpins clinical medicine in particular, is often characterized by variable, and small effects, certainly small relative to the noise in the measurements proposed. They favor rejecting the seductive promises to reduce randomness and complexity into a neatly dichotomous outcome (Gelman’s “uncertainty laundering”)
“A critical first step forward is to begin accepting uncertainty and embracing variation in effects (Carlin, 2016; Gelman, 2016) and recognizing that we can learn much (indeed, more) about the world by forsaking the false promise of certainty offered by such dichotomization.” (McShane, American Statistician 2019, 73:235-245)
Doug Altman offers (BMJ 1994, 308:283-284) the following solution:
“We need less research, better research, and research done for the right reasons.”